3 Ways to Ensure ESG Data Quality
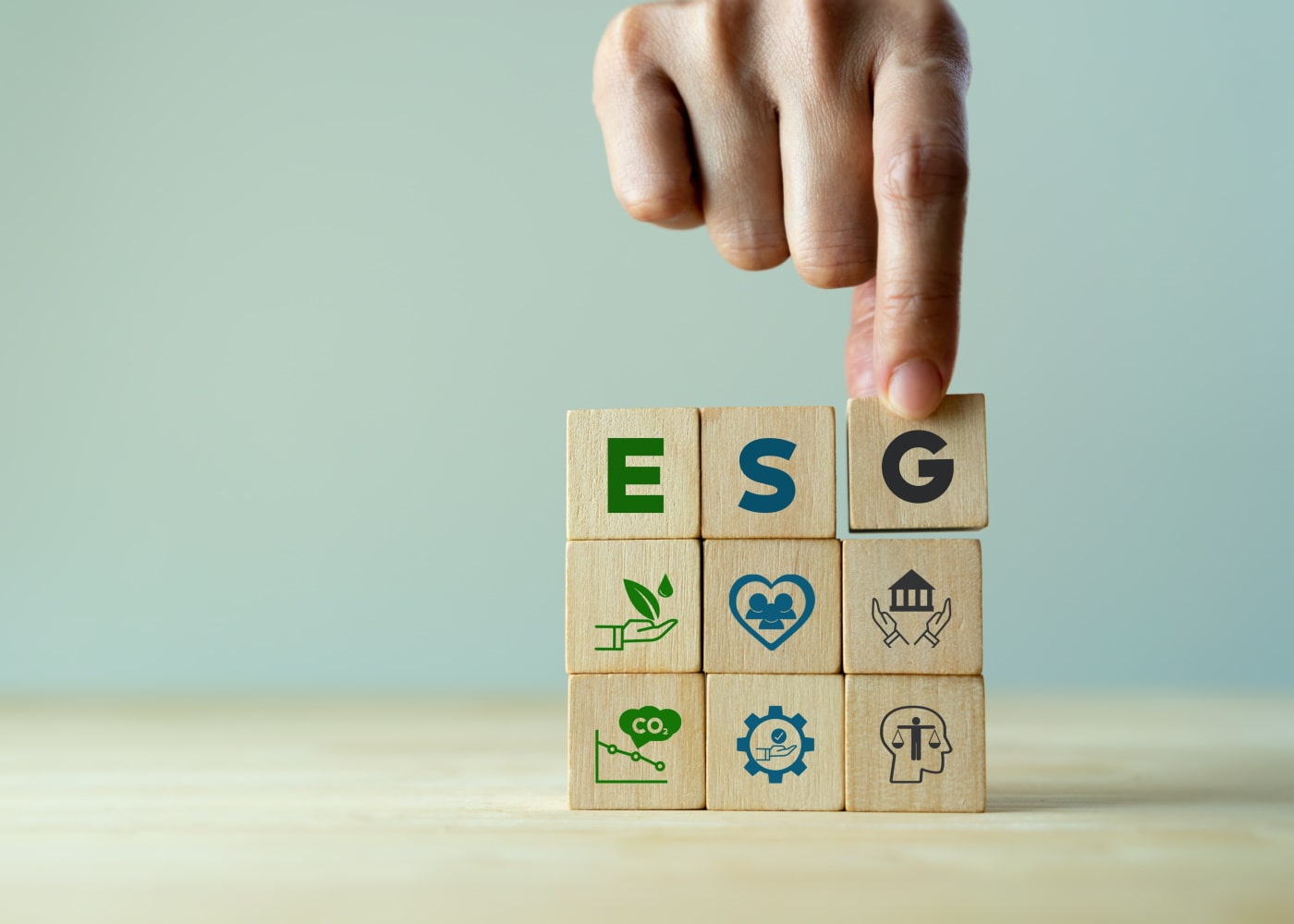
Previously, you could have suffered reputational problems if you disclosed inaccurate data. Now you can add non-compliance to the mix. Suddenly you have a legal problem, in addition to a reputational one, if your data is inaccurate. This means you must verify and ensure the quality of your ESG data.
Not all ESG data is the same. There are great differences in the complexity associated with various ESG metrics. The risk of inaccurate data therefore also varies greatly depending on the metric. For example, calculating carbon emissions across all your locations and all Scopes is not the same as calculating the amount of water consumed, or the percentage of executives and managers who are women, or the number of workplace injuries and fatalities. Data quality checks and procedures are needed for complex data points, such as carbon emissions, which also happens to be one of the most important ESG metrics.
Let’s look at how you can ensure data quality and reduce the risk of inaccurate data.
1) Automated Data Consistency Checks
The more data you collect, the greater the risk of having inaccurate data somewhere. The statistical odds of an error increase with the volume of data.Imagine you have sensors on hundreds of assets and equipment throughout your facilities, and you collect air emissions data at regular intervals (e.g. once a minute or every five minutes). You could end up potentially with millions of air emission data records, which you will aggregate and roll up at the enterprise level.
As the quantity of data increases, the risk of inaccurate data also increases. That’s why it’s a good idea to have automated validation rules and consistency checks through software.
For example, data values can be verified to see if they fall within an expected range. If there are outliers that deviate significantly from the expected range of values, they may be anomalies. These data records should be reviewed to see if something went wrong with the data capture or calculation.
Also, data values can be verified to ensure they correspond to what is expected based on historical values or best judgment. If there’s a big difference and it’s unexpected, then it could be a sign that there’s something wrong with the data.
With potentially thousands, if not millions, of emission data records, manually validating data is not an option. It has to be done through software that goes through all records and flags those that require attention.
2) Data Approval Workflows
Some types of metrics are fairly straightforward to measure. For example, the number of women on a company’s Board of Directors. So, as part of the ‘S’ of ESG, it’s relatively easy to calculate the percentage of women on your Board or executive management team.But other metrics are more complex, such as greenhouse gas emissions, chemical discharges, and other environmental metrics.
You will face many situations where you won’t simply enter data and stick it in a report. Based on the type of metric, you will want someone with more authority or knowledge to validate the data, or have at least a second person look also at the data.
Moreover, manual processes can create risks of human error. That’s why it’s better to use ESG softwareoffering configurable and automated workflows to ensure data is properly reviewed, validated, and approved.
Software-based automated data approval workflows help with auditability, which is one of the characteristics of investor-grade data. And stakeholders are increasingly demanding that ESG data be investor-grade.
In case of an audit, automated workflows allow you to find out exactly who entered a specific data value at a precise point in time, and who reviewed and approved the data.
3) Up-to-Date Data Integrations
As mentioned, the risk of inaccurate data increases with quantity. The greater the volume of data, the greater the risk of error. But there’s another factor that impacts data quality: data acquisition.Data entered by a person is more likely to have data quality issues than data sourced from another system, especially if it has already been validated in the external system. Typical issues with data entered by users include incomplete data, data in the wrong format, imprecise data, or even wrong values.
To reduce the risk of inaccurate ESG data, rely as much as possible on data integrations between your ESG software and external systems, such as HR systems, ERPs, asset management systems, data historians, process historians, etc.
Also, don’t forget to update the integrations. If either your ESG management softwareor the external system gets upgraded, be sure the integration is not broken or outdated.
In conclusion, when considering ESG software solutions, remember to look for features that help to ensure data quality. Failure to produce ESG reports is bad. But producing reports with inaccurate data is also bad, if not worse.
Related
Sponsored Content
About the Author
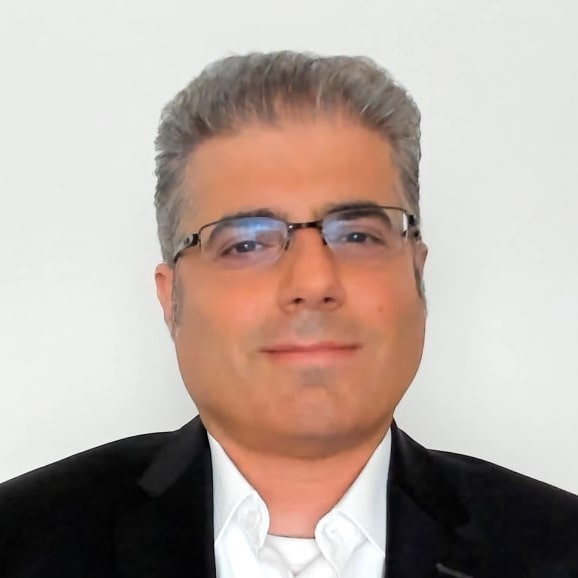
Jean-Grégoire Manoukian
Wolters Kluwer Enablon
Jean-Grégoire Manoukian serves as content thought leader for Wolters Kluwer Enablon, a leading provider of integrated software solutions that helps organizations protect worker safety, enhance sustainability, manage risks, stay compliant and identify
opportunities to elevate EHS, operational risk management, and ESG performance.