How Machine Learning is Opening New Possibilities for SDS Ingredient Indexing, Chemical Management, and EHS
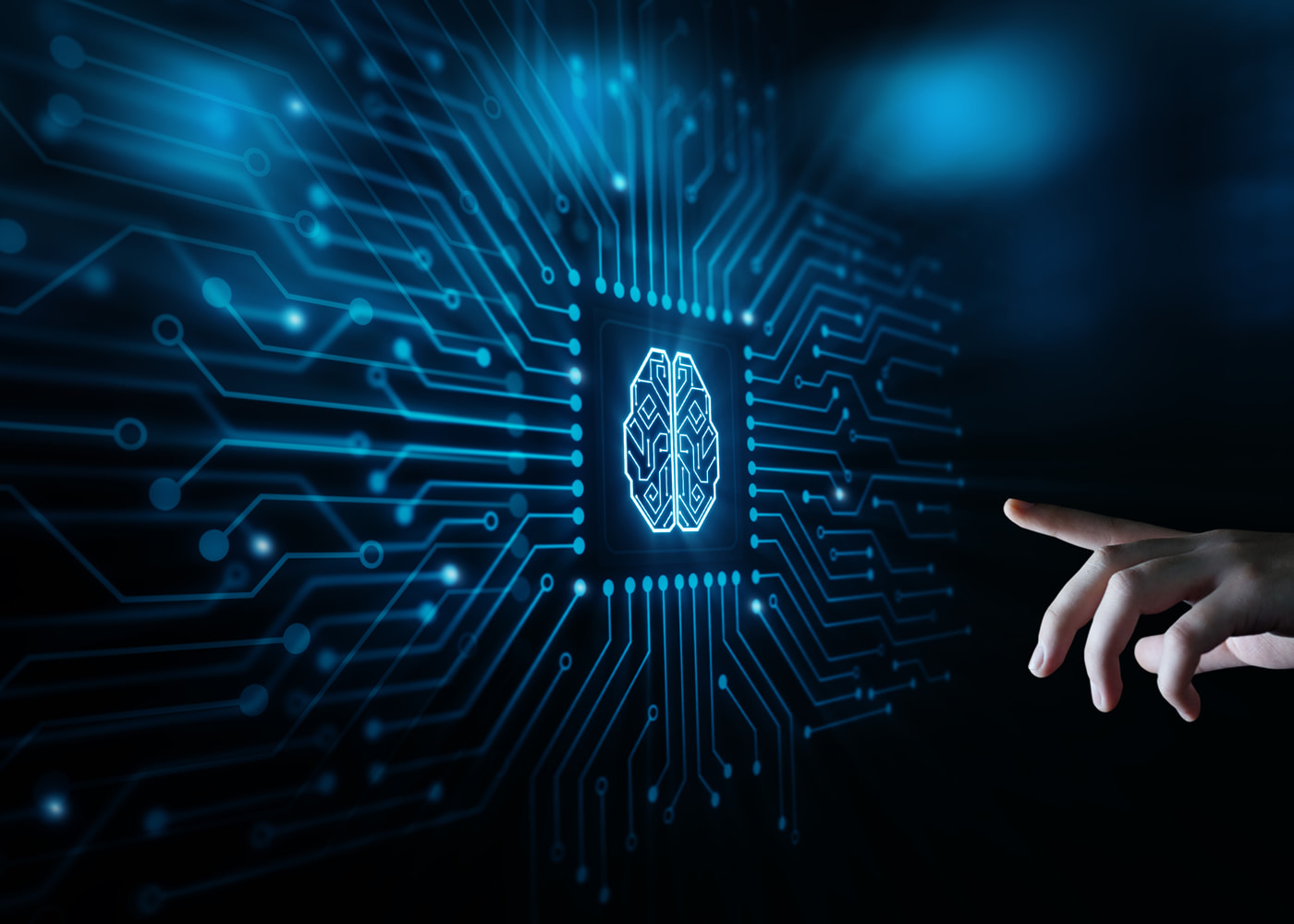
When combined with chemical management software, SDS indexing offers an added dimension of functionality above and beyond what can be achieved with paper or PDF-based SDSs alone. SDS indexing provides capabilities for digital categorization and search of SDS information based on the indexing criteria users choose, making it faster and easier to locate the chemical safety and hazard information when and where users need it.
SDS indexing also facilitates several other common chemical management and compliance tasks, including:
- Creating chemical container labels that include the specific SDS information you want to display, pulled directly from the SDS
- Providing detailed, real-time visibility of chemical inventory to better assess chemical hazards present in your workplace
- Building detailed chemical inventory reports to demonstrate compliance with hazard communication and chemical safety regulations
How is Machine Learning Being Applied to SDS Indexing?
Efforts to automate the indexing process using software have proven infeasible, difficult to scale, and prone to errors. This is because existing ‘rule-based’ software applications are limited in their ability to identify, extract, and index complex target information from digitized documents. Rule-based applications that search or match basic key words or key phrases in digital documents are common, but indexing complex information has so far proven difficult, especially when applied to variable file formats such as text versus image-based documents, or documents where information is presented in various formats such as tables and charts, in spreadsheet cells, free text, etc.SDS documents are a prime example of this complexity and variability. Within a single SDS, key information like chemical ingredients and compositions are typically represented using various structures, alphanumeric characters, symbols, and other technically specialized formats. Target information may fall under specific sections of the SDS, or within tables that could exist anywhere on SDS. Also, digital SDS file formats (PDF, .docx, .xml, etc.) can vary from manufacturer to manufacturer, making it very difficult from a technology perspective to automate the indexing of these documents, identify, extract, or analyze target information.
Emerging Machine Learning-Based SDS Indexing Technologies
Machine learning applications for SDS indexing continue to develop in the race to overcome the time and cost currently required by manual SDS indexing methods. VelocityEHS has very recently patented a new machine learning model designed to accommodate the complexity and variability of SDS information and formats. The model analyzes a digital version of an SDS and outputs a list of constituent ingredient identifiers along with their corresponding CAS numbers and weight percentages in a tabular format, which can be stored in a database or a file that can then be referenced by other applications such as a chemical management software system.The model applies a combination of Computer Vision and Natural Language Processing (NLP) technologies to index the SDS information present in tables, cells, or other variable formats. The model was trained and evaluated on an initial sample of 20,000 SDS documents and achieved a precision of 0.93 (93%). This level of precision is higher than existing rule-based software SDS indexing applications and significantly higher than the level achieved by manual indexing. The model continues to be trained on SDSs to improve this performance.
Chemical Management & Environmental Compliance
One of the most important types of information that employers need to index from an SDS is the name and the corresponding weight percentage of constituent ingredients within amixture. In some instances, product identifiers are indicated as trade names rather than proper IUPAC chemical names, and say nothing to describe the ingredient composition, weight percentages or concentrations, or the CAS identifiers which allow SDS users to reference the full SDS information for those constituent ingredients.Employers and chemical users need to be able to identify these constituent ingredients to assess the specific chemical hazards present in the workplace, assess the risks of those hazards, and implement appropriate controls in accordance with hazard communication standards like OSHA HazCom, Process Safety Management (PSM), and many others.
There are also applications for environmental compliance. Several EPA regulations like EPCRA Tier II and Toxics Release Inventory (TRI) require facility operators to report quantities of regulated chemicals stored or used in their facilities. Identifying the constituent ingredients of the hazardous products, as well as their weight percentages is the foundation for compliance with these requirements.
Industrial Hygiene (IH) Qualitative Exposure Assessment (QEA)
Automatically indexing SDS ingredient information has significant benefits for IH management, as well. Industrial hygienists need to be able to identify hazardous chemical that may be present in their workplaces, especially those which have established occupational exposure limits (OELs) so they can classify similar exposure groups (SEGs) and perform qualitative exposure assessments (QEAs) to monitor and control workplace chemical exposures.Machine learning SDS ingredient indexing can greatly simplify the process of identifying regulated substances and other potential stressors and enhance the ability of IH professionals to determine their corresponding amounts or concentrations. From there, ingredient information can be cross-referenced against a database of chemical ingredient PEL/OEL/TLV values to determine what SEGs, QEAs, and sampling activities need to be established to protect workers and maintain compliance.
Green Chemistry
Green chemistry is a key concern for chemical users pursuing environment, social, and governance (ESG) initiatives and looking to reduce safety and environmental risks posed by chemicals of concern in the workplace. Indexing SDS ingredient information to determine what risks are present is an important first step toward the ability to monitor and control those risks and ultimately prevent chemicals of concern from entering your workplace.The Future of Machine Learning in EHS
Machine learning is opening new frontiers across virtually every discipline, including EHS and ESG. At the same time, EHS professionals continue to transition away from paper-based methods and obsolete legacy software systems toward purpose-built EHS software solutions. Machine learning SDS ingredient indexing and its applications for chemical management, industrial hygiene, and green chemistry are only the start, and innovators in the EHS software industry continue to develop new machine learning applications that will further automate and simplify otherwise complex and time-consuming EHS management tasks.Related
Sponsored Content
About the Author

Greg Duncan, MELP, CSP
VelocityEHS
Greg Duncan is an EHS & Sustainability Content Expert at VelocityEHS. Prior to joining the company in 2016, Greg spent 6 years working with the National Marine Fisheries Service (NMFS) and Alaska Department of Fish & Game (ADF&G) aboard commercial
fishing vessels and at shoreside processing facilities in the Bering Sea and North Pacific to monitor fishing industry compliance with environmental laws and occupational health and safety standards. In his current role, Greg is focused on providing EHS
professionals with information and insights that help them to more effectively manage compliance and risk and build safer, more sustainable workplaces. Greg holds a Masters of Environmental Law & Policy from Vermont Law School, and a B.S. in Biology
from the University of Illinois.

Aatish Suman, MS
VelocityEHS
Aatish Suman is a Senior Machine Learning Scientist at VelocityEHS. He holds a Masters degree in Data Science from Syracuse University. Aastish has over five years of experience building machine learning solutions with expertise in Natural Language Processing (NLP) and document understanding.